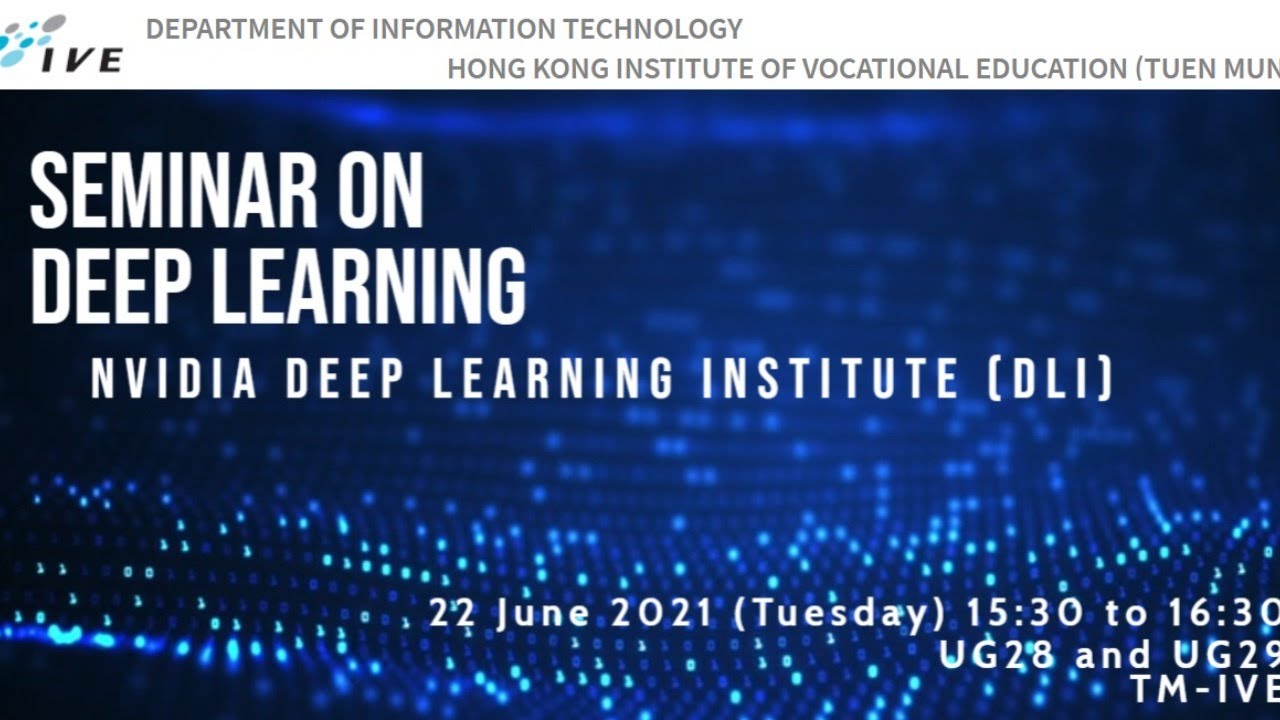
Deep learning uses state descriptions to calculate the output. Then, it determines what to act based on that information. This feedback is used to improve its deep network. Below is a discussion of the pros and cons of each. It is important to reward feedback for determining the outcome. Deep learning is a fast and powerful method that takes little time to learn. It can be used in a variety tasks, including robots and machine translation.
Unsupervised learning
There are many differences between deep learning and reinforcement-learning algorithms, and it is important to understand which one you should use. Deep learning is the most popular type of machine learning, while reinforcement-learning is a less popular option. Both methods have been successful in creating a wide range of high-quality products. You should be able to distinguish between them if you are a data scientist. Deep learning, which uses large data sets in order to create algorithms that learn through these data, is more efficient.
Contrary to reinforcement learning, reinforcement learning allows you to experiment with different actions and see which ones work best. Once the action is successful, the computer gets rewarded and the cycle continues. This means algorithms need to be independently developed for large numbers of iterations. If you are developing an autonomous car, for example, it is important that it doesn't crash into trees. Reinforcement learning algorithms are meant to make mistakes and reward the best.
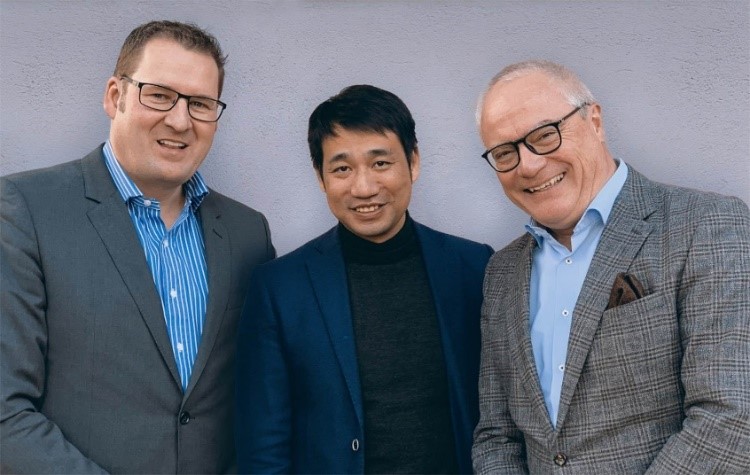
Reinforcement learning
Deep learning is a subset that of machine learning. It makes use neural networks to recognize patterns and make sense of data. It is commonly used for image recognition, natural language processing, and recommendation systems. Reinforcement Learning, on the other side, is a process that the agent learns through example. Deep learning techniques use large data sets and a lot of computing power. Both methods have their benefits and drawbacks, but they do share some key differences.
Reward-based teaching uses rewards to reinforce behavior. This is done by modifying the process until it matches the target's behavior. Deep learning employs reinforcement-based learning. It also uses data to improve performance. It is used to train robots for tasks. Regardless of the method chosen, it will be important to collect lots of data and apply the most effective algorithms for your needs. This will help you make the right decisions for your system as well as keep it running for many years.
Convolutional neural networks
Convolutional neural nets are artificial intelligence systems that learn from images. They represent an image using a tensor input. This input is transformed into a featuremap, also known by backpropagation, using an algorithm called backpropagation. Each CNN layer has a unique set of convolutional cores. The depth of the output volume controls the number of layers.
Convolutional neural network training is very similar to feedforward neural network training. The training process begins with random values, a tuple of images, and the classes the object belongs to. The network's output during training can be either 71% or 29% sure that the image is a cat/dog, or a mix of both. In a case like this, the number of classes required is two.
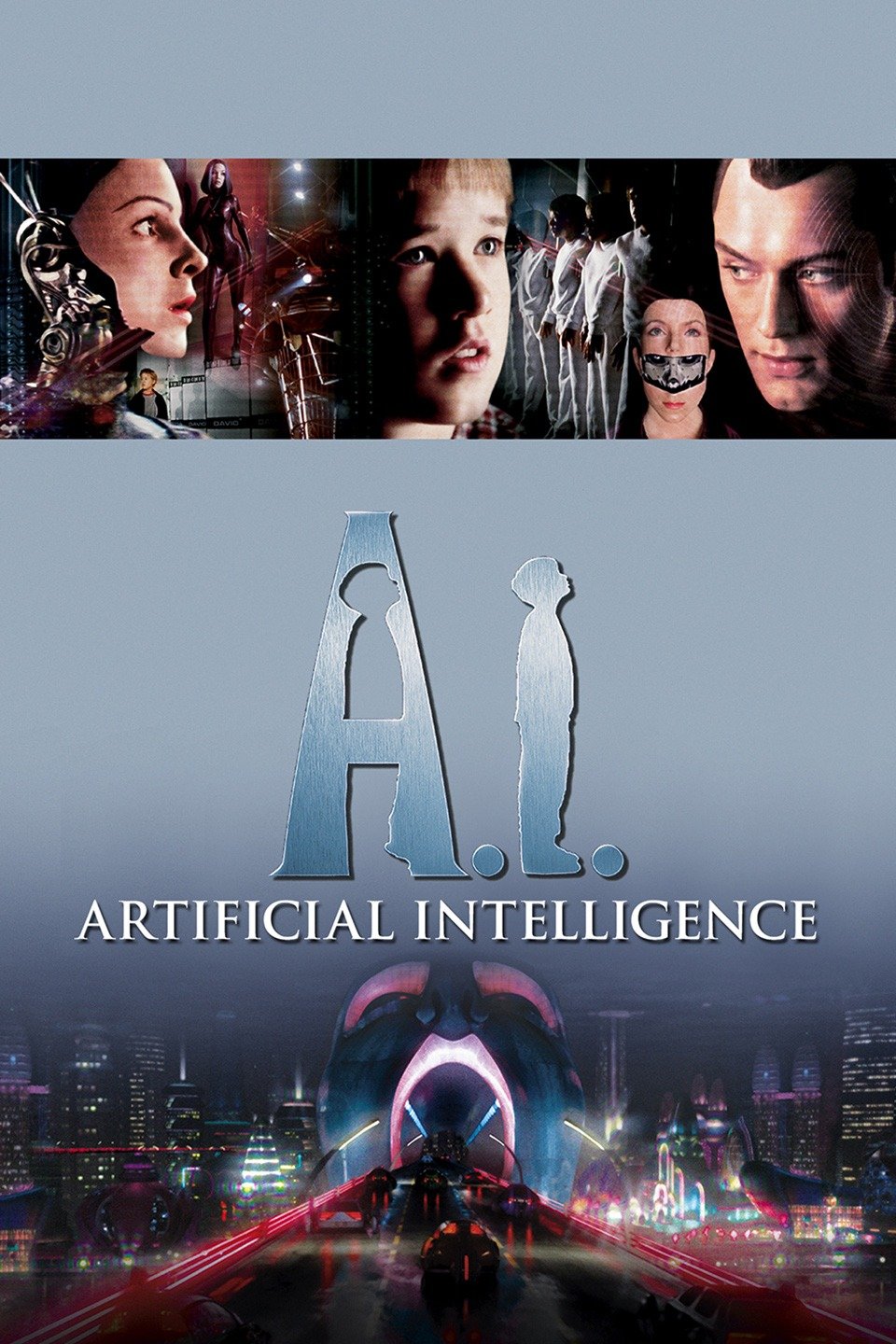
Deep learning applications
In many areas, deep learning as well as reinforcement learning are being used. While some of these fields are already using the technology, others are still in the research phase. This article will cover some of the more well-known uses of deep learning. Let's begin with virtual assistants. These virtual assistants, which can be activated by voice, are able to understand natural language commands and perform tasks on your behalf. They can also learn from your past experiences and develop new habits.
Computer vision, which is a branch of computer science concerned with the analysis of video streams and digital images, uses reinforcement learning and deep learning. Deep learning has played a significant role in this area of research. In computer vision, reinforcement learning has been effective in solving a variety of challenging problems, including image classification, face detection, and captioning. Reinforcement learning is also important in interactive perception. It is also used in other applications like object segmentation.
FAQ
How does AI impact the workplace
It will change our work habits. We will be able automate repetitive jobs, allowing employees to focus on higher-value tasks.
It will enhance customer service and allow businesses to offer better products or services.
This will enable us to predict future trends, and allow us to seize opportunities.
It will enable organizations to have a competitive advantage over other companies.
Companies that fail AI adoption are likely to fall behind.
What will the government do about AI regulation?
The government is already trying to regulate AI but it needs to be done better. They need to make sure that people control how their data is used. They must also ensure that AI is not used for unethical purposes by companies.
They also need to ensure that we're not creating an unfair playing field between different types of businesses. If you are a small business owner and want to use AI to run your business, you should be allowed to do so without being restricted by big companies.
Who invented AI and why?
Alan Turing
Turing was conceived in 1912. His father was a priest and his mother was an RN. After being rejected by Cambridge University, he was a brilliant student of mathematics. However, he became depressed. He discovered chess and won several tournaments. He returned to Britain in 1945 and worked at Bletchley Park's secret code-breaking centre Bletchley Park. Here he discovered German codes.
He died in 1954.
John McCarthy
McCarthy was born in 1928. He was a Princeton University mathematician before joining MIT. There he developed the LISP programming language. He had laid the foundations to modern AI by 1957.
He died in 2011.
Statistics
- According to the company's website, more than 800 financial firms use AlphaSense, including some Fortune 500 corporations. (builtin.com)
- Additionally, keeping in mind the current crisis, the AI is designed in a manner where it reduces the carbon footprint by 20-40%. (analyticsinsight.net)
- In 2019, AI adoption among large companies increased by 47% compared to 2018, according to the latest Artificial IntelligenceIndex report. (marsner.com)
- A 2021 Pew Research survey revealed that 37 percent of respondents who are more concerned than excited about AI had concerns including job loss, privacy, and AI's potential to “surpass human skills.” (builtin.com)
- While all of it is still what seems like a far way off, the future of this technology presents a Catch-22, able to solve the world's problems and likely to power all the A.I. systems on earth, but also incredibly dangerous in the wrong hands. (forbes.com)
External Links
How To
How to make Siri talk while charging
Siri can do many things. But she cannot talk back to you. This is due to the fact that your iPhone does NOT have a microphone. If you want Siri to respond back to you, you must use another method such as Bluetooth.
Here's how Siri will speak to you when you charge your phone.
-
Select "Speak When locked" under "When using Assistive Touch."
-
To activate Siri, press the home button twice.
-
Siri will respond.
-
Say, "Hey Siri."
-
Just say "OK."
-
Speak: "Tell me something fascinating!"
-
Say "I'm bored," "Play some music," "Call my friend," "Remind me about, ""Take a picture," "Set a timer," "Check out," and so on.
-
Say "Done."
-
If you would like to say "Thanks",
-
If you have an iPhone X/XS or XS, take off the battery cover.
-
Insert the battery.
-
Put the iPhone back together.
-
Connect your iPhone to iTunes
-
Sync your iPhone.
-
Set the "Use toggle" switch to On